
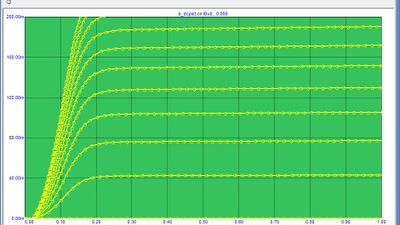
įinite dimensional operators use a deep convolutional neural network (CNN) to solve the PDE on a finite Euclidean Space. Photoacoustic imaging provides both structural and functional information that can potentially reveal novel insights into biological processes and disease pathologies. In addition to applying multispectral photoacoustic imaging to differentiate oxyhemoglobin from deoxyhemoglobin in breast cancer, ultrasound and photoacoustic tomography (US-OT) can reveal differences in lipids and collagen in breast fibroglandular tissue, providing more clinically meaningful insights for diagnosis. Multispectral photoacoustic imaging can be used for functional imaging, such as measuring blood oxygen saturation and metabolism in biological tissues. Specifically, in breast cancer detection, tumors have been successfully revealed by single-breath-hold photoacoustic computed tomography (SBH-PACT) without the need of ionizing radiation and exogenous contrast agents based on the higher blood vessel density characteristics associated with tumors. It has been applied for many preclinical and clinical imaging applications, such as small-animal whole-body imaging, breast and prostate cancer imaging and image-guided surgery. Photoacoustic imaging is a non-invasive hybrid imaging modality that combines the advantages of optical (e.g., high contrast and molecular specificity) and ultrasound (e.g., high penetration depth) imaging. Moreover, the FNO network was generalizable to the unseen out-of-domain test set with a root-mean-square error of 9.5 × 10 −3 in Shepp–Logan, 1.5 × 10 −2 in synthetic vasculature, 1.1 × 10 −2 in tumor and 1.9 × 10 −2 in Mason-M phantoms on a 64 × 64 computational grid and a root mean squared of 6.9 ± 5.5 × 10 −3 in the AWA2 dataset on a 128 × 128 computational grid. Results demonstrate that the FNO network generated comparable simulations with small errors and was orders of magnitude faster than the pseudo-spectral time domain methods (~26× faster on a 64 × 64 computational grid and ~15× faster on a 128 × 128 computational grid). Comparisons between the FNO network and pseudo-spectral time domain approach were made for the forward and adjoint simulations. In this work, we applied Fourier Neural Operator (FNO) networks as a fast data-driven deep learning method for solving the 2D photoacoustic wave equation in a homogeneous medium. Classical methods for numerically solving the photoacoustic wave equation rely on a fine discretization of space and can become computationally expensive for large computational grids. Simulation tools for photoacoustic wave propagation have played a key role in advancing photoacoustic imaging by providing quantitative and qualitative insights into parameters affecting image quality.
